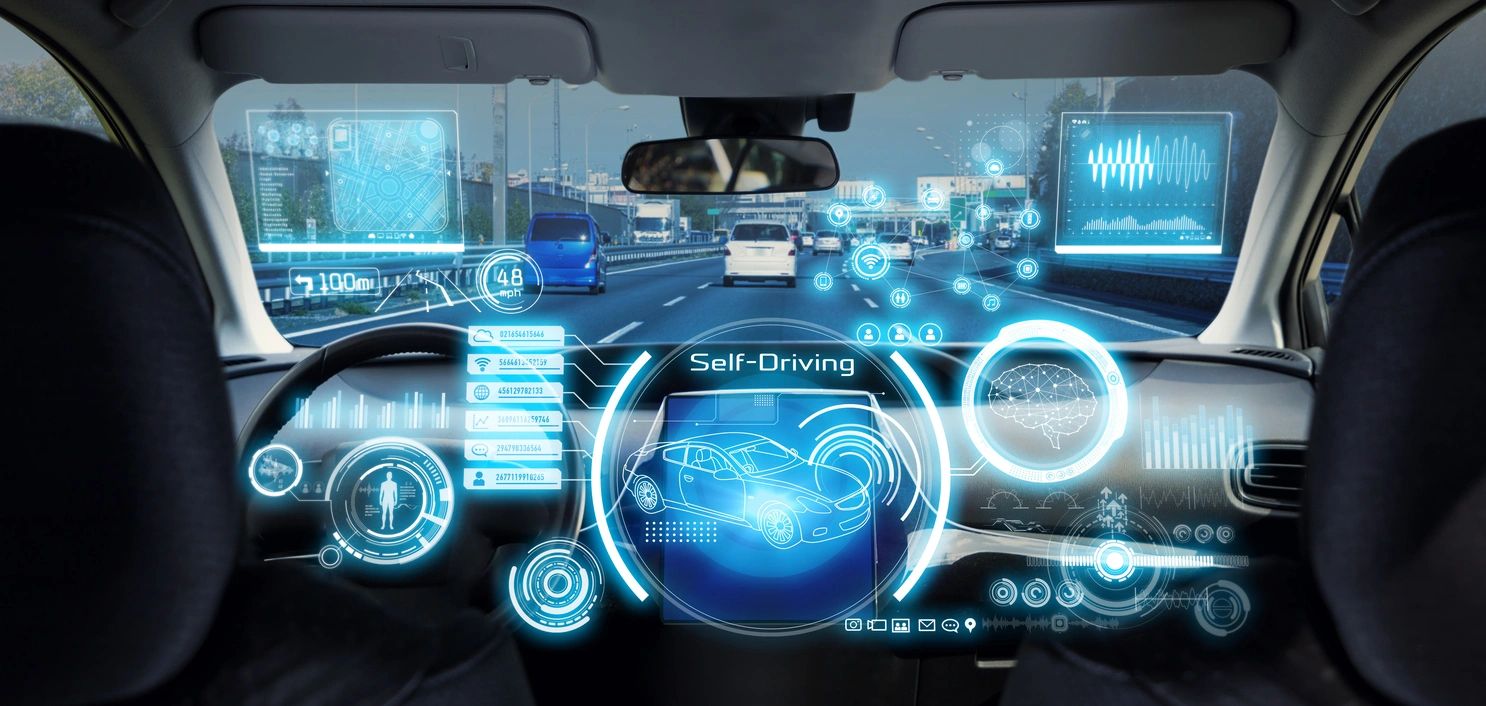
In this article by Professor John McDermid OBE FREng, University of York and Director, Lloyd’s Register Foundation Assuring Autonomy International Programme, explores the safety of Artificial Intelligence (AI) and the challenges in managing the risks associated with its development and use. Safe AI is described as being “free from unacceptable harm or risk due to the use of AI systems.” The article outlines the need to assess risks, especially in embedded AI systems (e.g., autonomous vehicles) and stand-alone decision-making AI systems. It emphasizes the importance of transparency, explainability, and meaningful human control over AI systems to ensure safety. It also highlights the pragmatic challenges of implementing these principles and suggests a domain-specific, multi-disciplinary, and principle-based regulatory approach to address evolving technology and risks effectively. Additionally, responsible innovation should consider the process, product, purpose, and people involved in AI development and use.
Prelude
Safety of Artificial Intelligence (AI) is a hot topic, with some saying that so-called foundation models pose an existential risk to humanity. AI has triggered diverse responses by different governments, including the EU[1] and USA[2], to try to manage the perceived risks of AI. The UK, perhaps uniquely, is seeking to balance innovation with risk[3]. But what is AI, what does safety mean, and how can we manage the risks without foregoing the benefits?
What is AI?
AI refers to computer systems able to perform tasks normally viewed as requiring human intelligence, such as image understanding and decision-making. Unlike “conventional” computer systems, AI is normally developed by training it to carry out a task by exposing it to data that provides examples. If it has been trained well on one set of data, the AI can then carry out the same task on data it has not seen before; for example, identifying previously unseen images as containing cancerous cells.
AI that addresses a single task, or a related set of tasks, is often referred to as “narrow” AI. In contrast, Artificial General Intelligence (AGI) would be capable of undertaking a whole gamut of human tasks, or at least intellectual tasks. So-called foundation models are trained on very large amounts of data and can be adapted to a wide range of tasks. Some see models such as ChatGPT[4] which can “converse” on a very broad range of topics, as a step towards AGI.
What is Safe AI?
In its simplest form, safe means ‘free from harm or risk’. However, there is never absolute safety in practice, and we need to consider acceptable levels of risk. Safe AI therefore means “free from unacceptable harm or risk, due to the use of AI systems”. The questions then become much more nuanced; what forms of harm, who or what can be risk-affected, and how are risks assessed?
What forms of harm and who is risk-affected?
We focus here on risks to individuals but note that societal and environmental harms also need to be considered[5]. Our focus is also on narrow AI since it presents a clear and present danger (as well as benefits), and there are already databases that track AI incidents[6]; whilst the potential harms of AGI arguably seem much more remote.
In considering forms of harm, we need to distinguish between embedded vs stand-alone types of systems. First, we consider AI which is embedded in a wider physical system, such as a robot or an autonomous vehicle[7]. Here, the most salient risks are to do with physical harm, and the risk-affected are those who use or come into close proximity to the system – in the case of autonomous vehicles (AVs) vehicle occupants and other road users; and potentially much of the population in the case of a domestic robot.
However, even such systems can pose threats to privacy, as the cameras on AVs will observe pedestrians, and AI will be used to track their movements. In general, a broad range of harms can arise from any one system – but we focus on physical harm for the purposes of this paper.
Second, there are stand-alone AI systems used in decision-making, or decision-supporting roles. Here the harms can be much more general, for example the inability to obtain a loan due to use of a credit-scoring AI-system, or not being considered for a job because of an AI-based CV screening system. These harms may arise from biases in the training data, which replicate and deepen existing human biases and societal inequity. Considering a CV-scoring system; if people from particular ethnic or socio-economic groups have historically taken a class of jobs, this historical bias can be reflected in the ongoing recommendations of the AI system. Such concerns are not just theoretical: the COMPAS system used to recommend prison sentences, based on risk of recidivism, has been shown to reflect racial biases[8].
Third, AI-based systems can also cause harm by being misused, abused or subverted. Well-known examples of system misuse to date (e.g., drones being flown near runways to close down airports[9]), can be attributed to human bad actors. However, enhanced with AI capabilities, such systems could become far easier to use and capable of inflicting harm on a broader scale; potentially with more serious consequences that are harder to counteract.
How do we assess risks?
Assessment of physical safety is a long-established discipline, dating back to the 1950s, with well-defined methods for quantifying risk. The introduction of software-controlled systems has led to a radical redefinition of safety processes, with the term “functional safety” being widely used. Here, quantifying risk is much more challenging, and it has become common practice to use a qualitative approach. A safety case[10] is often used to support a decision about allowing a system to be deployed.
The use of embedded AI requires a further radical revision of these approaches, although many of the principles and precepts still apply, and the safety case approach can be adapted to AI and autonomous systems[11]. These approaches build on safety engineering and functional safety experience, and reflect understanding of AI development processes (how training data is selected, and how models are developed and verified). However, due to the complexity of AI and the way in which the effectiveness and safety of systems can change in use, the use of pre-deployment safety cases is necessary but not sufficient. Through-life monitoring will be needed to ensure that systems remain safe; and to better identify undesirable trends to enable remedial action. This is already being reflected in some regulatory guidance (e.g., on Software as a Medical Device (SaMD)[12]), and is being considered in other domains, including autonomous driving.
But what about stand-alone AI systems, including those used for decision support? A common concern is the opacity of decisions or recommendations made by AI – a result of the complexity of the models, and how they are constructed. Consequently, there is a widespread call for transparency of AI, including access to training data. Proponents, including the Office for AI (OfAI)[13], believe that this should expose biases in training data and enable risks to be better managed; although this may be either impractical or unhelpful for foundation models. It is also common to seek explainability – generating explanations of the AI model as a whole or related to a particular decision. Such explanations can have value in a range of situations including pre-deployment assessment and for incident analysis[14]. Further, they can be used to support assurance[15] by, i.e., confidence in the capabilities and safety of the AI, and drawing together the safety case and explainability perspectives. This can also be seen in government guidance, e.g., from the Information Commissioner’s Office (ICO) on explaining decisions made by AI[16], which suggests the use of assurance cases.
We can extend the safety/assurance case approach into a broader consideration of ethical issues. AI can bring benefits as well as cause or contribute to harms – and the UK Government’s approach seeks to balance the two. Medicine already grapples with such issues – for example giving a patient chemotherapy causes harm, but with the intended benefit of treating cancer. Such concerns and conflicts have been encoded in a framework of biomedical ethics[17]. It is possible to produce assurance cases for AI by adapting the principles of biomedical ethics and supporting them with transparency, to provide an ethics assurance framework[18] to assist in responsible innovation.
Principles
Reflecting on the above, and particularly the adapted biomedical principles, we can now articulate 5 (+1) principles for the safe use of AI:
- Identify the benefits and the beneficiaries.
- Understand the harms and identify the risk-affected.
- Ensure meaningful human control over the stand alone or embedded AI system, (note: if direct human control is not possible, e.g., due to the speed of response required, then that control has to be exercised through assurance and regulatory processes[19]).
- Ensure transparency of the AI system itself and the assurance evidence.
- Seek to balance the risks and benefits across the beneficiaries and risk-affected so that, for example, all risk-affected individuals also receive benefits to ensure fairness.
This is broadly in harmony with the OfAI’s principles (fairness, accountability, sustainability, and transparency), with the identification of benefits, harms and human control giving a more explicit basis for judging fairness. Sustainability is addressed by considering societal and environmental benefits and harms, so this structure has the flexibility to integrate some of the OfAI’s principles. It will also be necessary to clarify notions of responsibility and accountability which requires consideration of legal, ethical and technical perspectives[20] and this might lead to enhancement of the above principles.
As noted above, the capabilities (and hence benefits and harms) of AI systems can change over time, even without learning in operation. Thus, the “+1” principle is to monitor systems in operation and to identify the need to take recourse if what was assessed to be safe, fair and acceptable on initial deployment ceases to be so, or there are undesirable incidents. Although this sounds simple, it is challenging in itself and needs to be enabled by good system design[21]. There will also need to be collaboration between developers and those investigating incidents.
Pragmatics
As Tolstoy noted: “It is easier to produce ten volumes of philosophical writings than to put one principle into practice”. Yet, system developers, policy makers and regulators have to put principles into practice. How can this be done?
First, there is the need to recognise that judgements about fairness, acceptability of risk, etc.,. are domain specific. For example, the risk of harm from an AI-based decision-support system that might be acceptable or tolerable due to the attendant benefits in a clinical setting, might not be tolerable in a domestic setting without healthcare professionals to mediate the output. Thus, although there is merit in having generic principles, thus achieving consistency in policy-making across government, there is also a need for domain-specific rules and regulations. This implies that the responsibility for dealing with AI must remain predominantly with the existing regulators – even if they need to be supported by expertise in central government.
Second, judging fairness requires engaging all relevant stakeholders, beneficiaries and the risk-affected (or their representatives)[22]. There is also a need to take a multi-disciplinary approach as the knowledge to assess risks and benefits does not lie within a single discipline. For example, a Centre for Data Ethics and Innovation (CDEI) report on autonomous vehicles[23] brought together a multidisciplinary team and produced some actionable recommendations including structural changes in governance, and covered issues such as privacy, safety cases and in-use monitoring. Whilst the recommendations are quite demanding, this shows what can be done to produce actionable guidance for a specific sector.
Third, regulatory frameworks should follow the long-established UK tradition where the primary legislation is principle- and risk- or goal-based, supported by more specific guidance where appropriate. Given the pace of change of technology, trying to be prescriptive seems impracticable – the technology will have evolved well before the legislation has been passed[24]. The Law Commission’s recommendations on self-driving vehicles[25] have brought helpful clarity (e.g., on what it means for a vehicle to be self-driving) and indicated where additional guidance will be needed. As well as being a good example of principles-based regulation, it also reflects the first two points; it is domain-specific and it has also been developed following considerable multi-stakeholder and multi-disciplinary consultation.
Fourth, there is a need to identify and research some of the deep problems that could impede effective regulation. For accountability to be legally enforceable, there needs to be a notion of causality that ties the adverse impacts of the AI to the developer (where that is appropriate). The EU has tried to address this by introducing the presumption of causality – in a legal context it is presumed that the AI led to harm, hence the developer is liable. However, it is far from clear that those definitions will always work in practice, and this is likely to be an important area of study. The OfAI principles include transparency which will often include explainability. But will it be possible for a self-driving vehicle to provide useful explanations as it drives? Approaches such as Digital Commentary Driving (DCD)[26] may make it possible for a self-driving vehicle to provide useful explanations as it drives, but further research is needed to know whether or not this will be practicable, and whether its merits lie more in pre-deployment assurance or in incident investigation.
Finally, it is likely that there will be a need to preclude certain uses of AI, and again, the EU identifies certain classes of systems which are unacceptable. Rather than encoding such things in legislation, there would be merit in an assurance-based approach, reflecting the principles identified above. If the benefits outweigh the risks and there is sufficient human control, then the system could be deployed; if not, then the system should not be used. Whilst this approach requires more work at the outset, it provides for flexibility as technologies evolve, use cases mature and assessment methods evolve. The principles of allowability remain, but the parameters can change without lengthy legal intervention.
In complex situations, actionable principles trump prescriptive legislation.
Postlude
Guidance on responsible innovation is often framed as 4Ps: process, product, purpose, people. What has been outlined above is product-focused, but also embraces purpose (via considering benefits) and people (stakeholders and risk-affected). The process is reflected in the assurance case approach – but that is not enough. The processes might, for example, employ people annotating images or doing other low-level tasks preparing data for training AI models. These individuals may be poorly paid and work in terrible conditions[27]. Thus, for a complete approach to responsible innovation some additional precepts are needed for responsibly managing supply chains. However, the above principles and pragmatics give a starting point for addressing the core elements of responsible innovation, as well as helping achieve safety of AI, thereby enabling the benefits of AI to be realised whilst avoiding or reducing its harms.
Further acknowledgements:
The author wishes to thank the following University of York colleagues for their contributions to this article: Dr Rob Alexander, Professor Radu Călinescu, Professor Ibrahim Habli, Dr Colin Paterson and Dr Zoe Porter.
[1] https://artificialintelligenceact.eu/the-act/
[2] https://www.whitehouse.gov/ostp/ai-bill-of-rights/
[3] https://www.gov.uk/government/publications/ai-regulation-a-pro-innovation-approach/white-paper
[4] https://openai.com/blog/chatgpt
[5] McDermid, J.A., Burton, S. and Porter, Z., 2023, February. Safe, ethical and sustainable: framing the argument. In The Future of Safe Systems: Proceedings of the 31st Safety-Critical Systems Symposium, 2023 (pp. 297-316).
[6]https://incidentdatabase.ai/apps/discover/?display=details&is_incident_report=true&page=1&sortBy=instant_search-en-featured
[7] The Law Commission used the term “automated vehicles” (https://www.lawcom.gov.uk/project/automated-vehicles/ ); the intent here is any system where the occupants do not have to engage in the driving task.
[8] https://www.propublica.org/article/how-we-analyzed-the-compas-recidivism-algorithm
[9] T Burridge. ‘Sustained’ drone attack closed Gatwick, airport says. BBC News, 20 February 2019. https://www.bbc.co.uk/news/business-47302902 .
[10] An argument, supported by evidence, that a system will be acceptably safe for use in a defined operational context.
[11] https://www.assuringautonomy.com
[12] https://www.gov.uk/government/publications/software-and-ai-as-a-medical-device-change-programme/software-and-ai-as-a-medical-device-change-programme-roadmap#wp-4-post-market (especially WP4)
[13] https://www.gov.uk/guidance/understanding-artificial-intelligence-ethics-and-safety
[14] McDermid, J.A., Jia, Y., Porter, Z. and Habli, I., 2021. Artificial intelligence explainability: the technical and ethical dimensions. Philosophical Transactions of the Royal Society A, 379(2207), p.20200363.
[15] Jia, Y., McDermid, J.A., Lawton, T. and Habli, I., 2022. The role of explainability in assuring safety of machine learning in healthcare. IEEE Transactions on Emerging Topics in Computing, 10(4), pp.1746-1760.
[16] https://ico.org.uk/for-organisations/uk-gdpr-guidance-and-resources/artificial-intelligence/explaining-decisions-made-with-artificial-intelligence/annexe-5-argument-based-assurance-cases/
[17] Beauchamp, T.L. and Childress, J.F., 2001. Principles of biomedical ethics. Oxford University Press, USA.
[18] Porter, Z., Habli, I., McDermid, J.A. and Kaas, M., 2023. A principles-based ethics assurance argument pattern for AI and autonomous systems. AI and Ethics, pp.1-24.
[19] https://assuringautonomy.medium.com/human-control-of-ai-and-autonomy-the-art-of-the-possible-66779846e1d8
[20] https://tas.ac.uk/responsibility-project-ar-tas/
[21] The software “bug” that led to the December 2014 failure of UK Air Traffic Control was identified in about 45 minutes (https://www.caa.co.uk/media/r42hircd/nats-system-failure-12-12-14-independent-enquiry-final-report-2-0-1.pdf; it seems unlikely the same could be achieved with AI, without very careful design, or maybe not at all.
[22] Townsend, B., Paterson, C., Arvind, T.T., Nemirovsky, G., Calinescu, R., Cavalcanti, A., Habli, I. and Thomas, A., 2022. From pluralistic normative principles to autonomous-agent rules. Minds and Machines, 32(4), pp.683-715.
[23] https://www.gov.uk/government/publications/responsible-innovation-in-self-driving-vehicles
[24] The EU’s approach in their AI to defining AI by listing types of technology seems questionable, at best.
[25] https://s3-eu-west-2.amazonaws.com/lawcom-prod-storage-11jsxou24uy7q/uploads/2022/01/Automated-vehicles-joint-report-cvr-03-02-22.pdf
[26] https://www.bsigroup.com/en-GB/about-bsi/media-centre/press-releases/2021/june/digital-commentary-driving-the-new-safety-technique-that-can-help-put-automated-vehicles-on-our-roads/
[27] https://www.theverge.com/features/23764584/ai-artificial-intelligence-data-notation-labor-scale-surge-remotasks-openai-chatbots